AI has been known in specialist circles since the 1990s. However, it only became widespread with the publication of ChatGPT in November 2022. Most companies quickly realise that AI is not the next cow to be driven through the village. And yes, AI is still a relatively young technology that is still maturing. But it will mature very quickly and there are already various use cases in which AI is already delivering massive added value. And what is impressive about AI is the speed at which it is developing. If you are not careful, you run the risk of being overtaken left or right very quickly.
Many companies now have their own digital strategy and experience with digitalisation. As artificial intelligence is now the next big step, it is all the more important to define and implement an AI strategy for your own company as part of your own digital strategy.
Why define an AI strategy for your company?
For most companies, AI, and generative AI in particular, came out of nowhere. AI offers a wide range of potential applications, for example in the optimisation of processes, the provision of knowledge and so on. However, there are also risks associated with the use of AI. If employees use “any” AI tools from the internet and upload documents there, the sacred company knowledge can quickly be used to train AI models and in future be given to competitors as a possible answer to their question.
To avoid this and define a safe approach to AI, companies should define a strategy for dealing with AI. In addition to safe use, it is particularly important to gain experience and build up expertise. The technology is developing so quickly – those who are not able to test will not understand the technology and will quickly be left behind in this area.
What does the implementation of an AI strategy require?
On the one hand, there is the legal framework, which should be mapped in your own AI strategy. On the other hand, it must of course also be taken into account that not all AI is the same. There needs to be a framework for understanding, trialling and testing. And, of course, communication is also part of this, because for many employees, the word “artificial intelligence” is associated with a lot of dangerous half-knowledge, sometimes also through headlines from the press. It is therefore important to consider the following tips:
1. Define goals
What is to be achieved by introducing the AI strategy? What is it about in the short, medium or long term? Clarifying these overarching goals helps to prioritise and also to identify use cases.
2. Provision of resources
Unfortunately, an AI strategy is not realised in one night. However, in order to successfully define it, interdisciplinary teams are needed that also look at things from the user side, among other things. The implementation and development of expertise should be clearly anchored in the objectives (ideally with time investment) of the employees as well as a budget for pilots, external support, etc.
3. Communication
For many employees, AI sometimes has negative connotations – “AI will take their job”, “I can’t use AI because we’ll lose our expertise”, etc. There are many assumptions, some justified, some unjustified, which are due to a lack of understanding of the technology. It should therefore be clear from the outset what the project is and under what conditions AI may be used. Ideally, the IT or digital department should provide a list of “safe” AI applications that can be used by employees without hesitation. This list does not have to refer exclusively to paid tools, but can also include free tools.
To break this information down further, certain use cases or data that may be used as input can also be defined.
4. Test data for pilots
AI is often inexplicable. In order to understand the technology, employees must be given the opportunity to experiment with solutions and generate test data sets (machine data, presentations, templates, etc.) with little effort. In order to streamline approval processes and quickly build up expertise, such data sets should be defined in advance so that employees can quickly demonstrate results. Such initial demonstrators can then be further developed into real pilots.
5. Prioritise use cases
AI is basically a means to an end. Various use cases must therefore be defined. We use the Now, How, Wow framework to prioritise these.
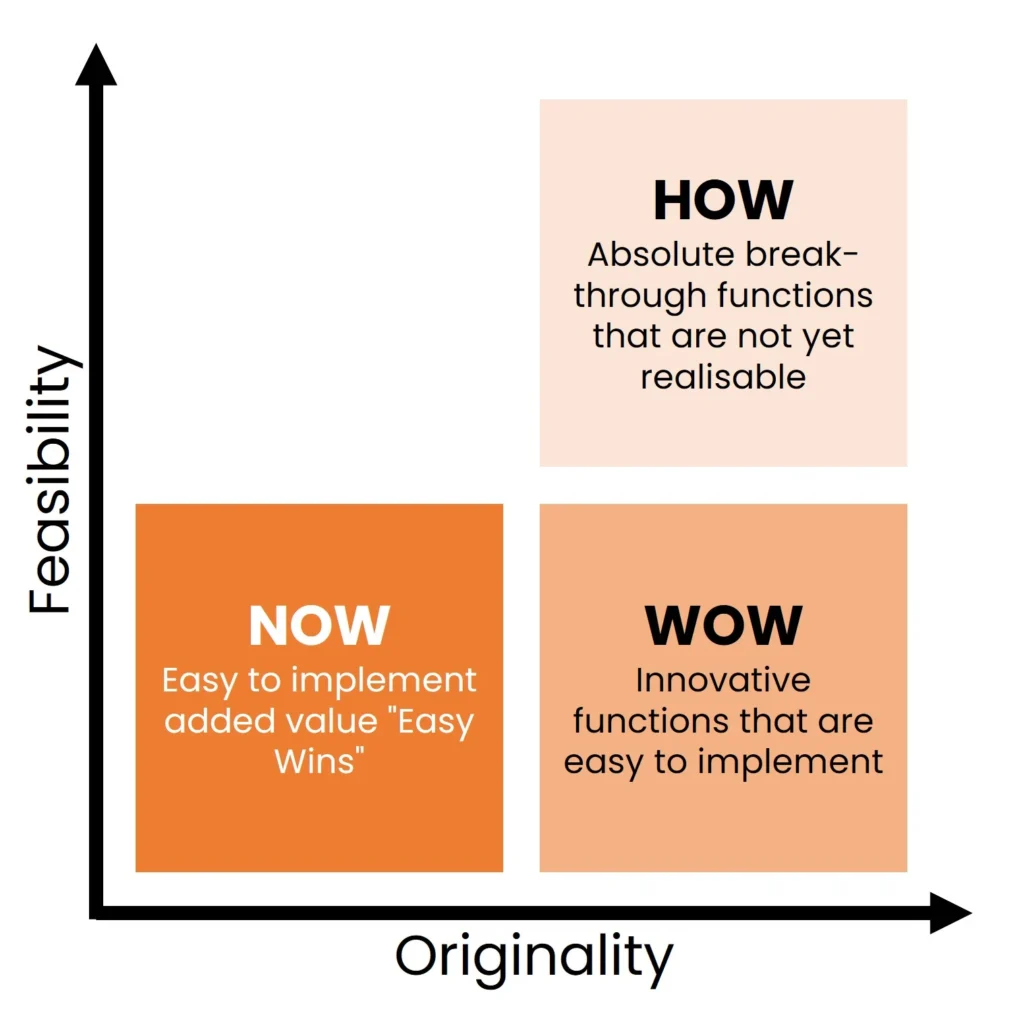
Figure 1: Example of how to prioritise use cases of generative AI
In order to prioritise use cases, especially in terms of feasibility, we need to build up an understanding, which leads us to the sixth point:
6. Technology understanding
In order to set realistic expectations for AI, you need to understand how AI works. We often see companies come to us with “unrealistic” expectations because the technology is simply not advanced enough yet. In the Now, How, Wow framework, for example, this would be a Wow use case – so it should be saved for later. Try to solve the Now use cases to achieve the aha effects and not cause frustration.
7. Change Management & Education
One thing is to understand the technology. The other is to apply it and realise the added value. A learning space must be created for employees so that they can continuously learn from each other. Only if success stories and “aha” moments are regularly shared with the team can the integration of AI into everyday life be truly sustainable.
Integrating AI into everyday life
AI will not arrive with employees overnight and its use will require training and time. Employees need to gain their own experience and they need this time – as was the case with teams in many companies. If acceptance is not so high at the beginning, but the potential is seen, then you should think three times before cancelling a pilot or whether it would be better to continue and work together on improvements.
Standard solutions vs. customised solutions for artificial intelligence
Most use cases affect a large number of companies and can be used by many employees. AI therefore does not always have to be expensive and require high-priced pilots.
However, hosting an AI (especially if it is an AI based on large language models) on premise usually makes little sense or only makes sense for larger companies (KRITIS). The hardware resources and expertise required to maintain them are too great. Instead, there are solutions such as amberSearch, which as a standard system (and usually as a managed service) provide SMEs with a GDPR-compliant solution. We pay particular attention to ensuring that companies can retain their independence with us by functioning as a cross-system tool.