Gaining experience with AI is an important priority for many companies this year. However, the question is where to start and which use cases to prioritise.
This blog post focuses in particular on the use of generative AI in combination with internal company data
, for example to make in-house expertise more accessible. Some companies have already defined an AI strategy and are now considering how they could go about implementing it. Of course, the first step is to identify AI use cases.
Identifing AI use cases
The following definition is used to identify an AI use case:
“An AI use case is given when an AI-based software solution solves a problem that cannot be solved equally or better with conventional software.”
Depending on the use case, the use of AI can be more resource-intensive than the use of conventional software. AI should therefore have a clear added value in an application compared to a conventional solution. One example of this is the use of AI in internal company searches.
An AI use case always requires an overriding goal, which is not achieved to a satisfactory degree. One example could be increasing efficiency in a company. In order to increase efficiency, a company has defined a digital strategy. This in turn gives rise to various sub-projects or interim goals that need to be optimised. An AI use case could therefore result from the digital strategy.
However, an AI use case could also result from demographic change. The baby boomer generation is retiring and too few people are coming along. This means that, on the one hand, there is a lot of expert knowledge in the heads of long-standing employees that needs to be digitised and, on the other hand, the already digitised know-how needs to be made available to a new generation of employees who, at least digitally, think differently and have different expectations. Another use case could arise from the fact that the resulting shortage of skilled labour means that the same or more work has to be done with fewer human resources. Incidentally, we have described in this blog article what the concrete definition of an AI use case can look like using an example.
As you can see, it’s always about solving problems. If you want to capture problems in a structured way, you can use the JTBD framework.
How the JTBD framework works
JTBD stands for Jobs-to-be-Done and is a question framework that consists of 3 steps:
- Jobs to be done: The first step is to understand the user’s task. To do this, you should ask as neutrally as possible why a person is working on certain topics, what their goals are, etc.
- Pains: The second step, the Pains step, is about finding out the reasons why something is not working as well as expected or what is preventing the person from achieving the given goals.
- Gains: The third and final step is about the gains. What would the situation look like if the problem were solved? What should improve in the process/result as a result of the solution and what are the added values?
This process can be used to identify what the challenges are in the workforce and to build on this to identify the biggest pain points with the greatest added value.
Using AI to identify use cases
After applying the JTBD framework, there should be a whole series of problems that were just waiting to be solved. On the one hand, solutions can of course be searched for directly in Google. Alternatively, AI tools such as ChatGPT can of course also be consulted to find out how the problems can be solved and for which use cases it potentially makes sense to use AI.
Prioritising AI use cases
There are 2 evaluation factors for prioritising the various use cases developed:
- Business impact/ROI
- Technical feasibility
Business impact/ROI
In order to calculate the business impact or ROI, knowledge of various topics is required. Roughly speaking, these are the following topics:
- How high is the effort in the status quo without AI and how great is the pain? Of course, the pain can be measured in different directions (quantitative, qualitative or financial, employee satisfaction …)
- What would change through the realisation of the AI use case and how high is the necessary investment (financial, know-how, time, …)?
- How does the implementation help to achieve the defined goals (short, medium, long term)?
In addition, things such as risk matrices help in the evaluation of such tasks.
Technical feasibility
Various factors should be taken into account when assessing technical feasibility. On the one hand, a certain technical understanding is necessary in order to be able to make a realistic assessment and not be solely dependent on the statements of the providers. On the other hand, frameworks such as the Now-How-Wow Framework can help.
How the Now-How-Wow Framework works
In order to assess the feasibility of use cases, the AI use cases and potential solutions are categorised into 3 categories: Now, How and Wow.
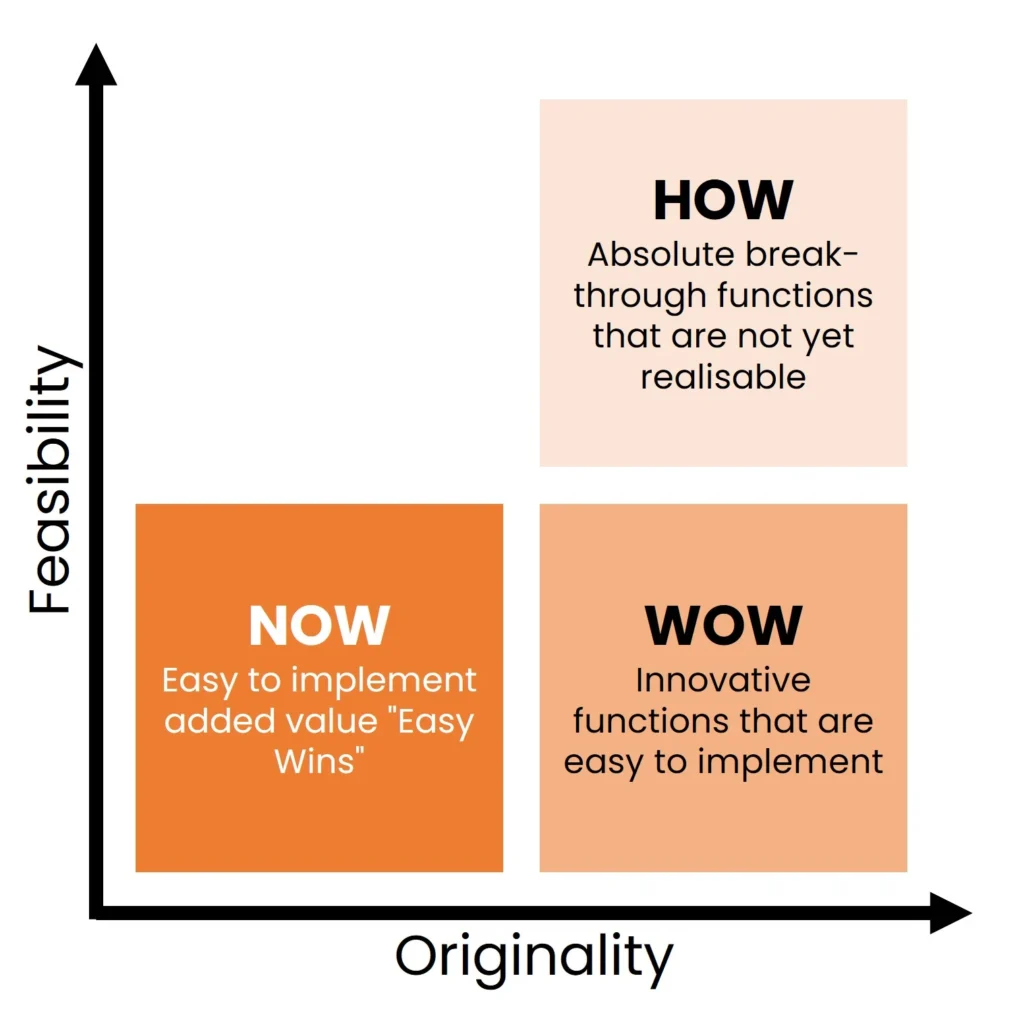
Framework Now-How-Wow
- Now stands for use cases that can be implemented directly and without major (technical or resource-based) problems.
- Wow stands for use cases that can be realised through technological advances with acceptable risks/resource expenditure. As the implementation of such use cases is particularly desirable (e.g. use of generative AI with in-house expertise), they are often marketed externally in order to demonstrate the joy of innovation.
- How stands for use cases that are not yet technically feasible and still require some research and development before they become wow use cases.