Generative AI Search is a software category that combines the disciplines of search and generative AI. Generative AI search is applied either to data on the internet or to internal company data – but then in combination with enterprise search and generative AI.
Table of Contents
Data volumes are growing ever faster and are usually distributed across different systems. It is therefore becoming increasingly difficult for users to find the right information. This is precisely where the combination of generative AI and an intelligent search can help.
An intelligent search as the basis
There was a breakthrough in AI research in 2017. So-called transformer models were able to search large volumes of data quickly and efficiently on a semantic basis. Where previously keyword-based search algorithms – supported by NLP-based functions – were the state of the art, it is now possible to evaluate information based on the semantic meaning of the content. Sounds relatively technical, which is why we have published further details on various search technologies in this blog article.
These intelligent searches are based on large language models that specialise in retrieval, i.e. the evaluation of information based on similarities. In order to enable a fast and high-performance search, an index is first created, which must be used to map access rights on the one hand and information such as changing content and various file formats on the other.
If a user asks a question, the retrieval model automatically searches for suitable hits and lists them – as is familiar from web search engines, for example. If such logic/technology is used in a company, this is known as enterprise search.
Generative AI as a personalised extension of the search
There have been few technologies in recent years that have triggered as big a wave as generative AI. The end of Google’s dominance has often been predicted and there are currently no serious signs of the top dog changing hands. What is striking, however, is that Google is also working hard to integrate generative AI into its search. Generative AI makes it possible to answer a question as continuous text and not just in a list of results. This enables significantly more personalised results and increases user-friendliness, for example by giving users the opportunity to ask follow-up questions or specify questions more precisely.
The fusion of search and generative AI
A Retrieval Augmented Generation (RAG) system is usually used to combine search and generative AI. This is how a RAG system works:
- Retrieval – When a user submits a query, a search is first carried out in the previously created index for semantically relevant content. The results are listed – sorted by relevance.
- Augmentation – The matching results are passed to a generative AI. Basically, the prompt looks like this: “The user has asked the following question “X?”. These are results 1, 2, 3, … . Use the results to formulate an answer to the question.
- Generation – The generative AI now uses the results and information from the prompt to answer the question.
The fusion of these two techniques becomes particularly sustainable if this technology is well integrated into existing systems – e.g. the Internet or IT infrastructures.
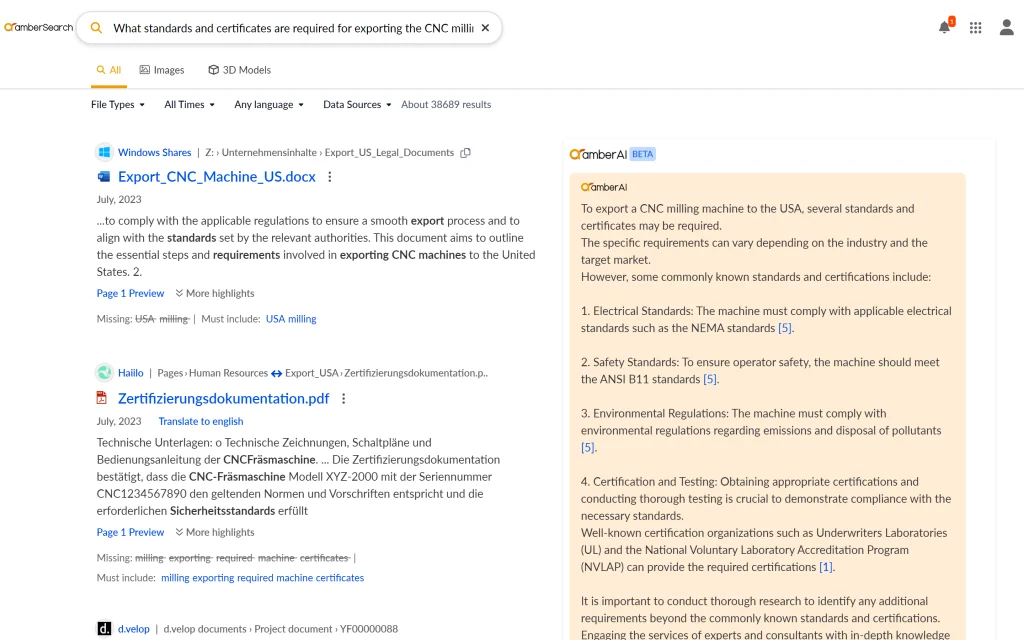
Example of a generative AI search from amberSearch. The search is shown on the left, the personalised and generated response on the right.
Areas of application for Generative AI Search
The main areas of application for Generative AI Search are on the Internet and when used with internal data.
Generative AI Search on the Internet: Potential and challenges
There are many challengers for Google on the internet, such as Bing Chat, you.com and Perplexity, which are currently trying to revolutionise web search. The user experience is undeniably better and shows significantly improved usability.
However, one of the challenges faced by these providers is that traditional business models, such as displaying results for AI-generated answers, are much more difficult than displaying a list of search results. Another challenge for the challengers is that users are used to providers such as Google and Google is very well integrated into everyday user life thanks to various free services.
Internal company data and generative AI search
The second major use case of generative AI search is the combination with internal data. After all, the management of internal expertise is becoming increasingly difficult, as both the volume of data and the number of data silos in companies are increasing massively.
There are various use cases for a generative AI search in a company. For example, our customer Zentis has used our generative AI search to make the expertise that has accumulated over the years in different languages and at different locations quickly and easily accessible.
The ENTECCOgroup uses a generative AI search to make the differently structured expertise quickly and easily accessible to new employees after a merger.
And DB Regio, for example, uses a generative AI search to answer customer service questions more quickly.
The advantages of using generative AI and enterprise search are clear:
Existing expertise can be utilised significantly better and more efficiently. Generative AI Search is therefore also a possible solution in AI-based knowledge management.
Security and data protection aspects of Generative AI Search
One of the most important issues when using a generative AI search is ensuring that expertise is not lost and that existing laws and security guidelines are taken into account.
At amberSearch, we have therefore introduced various security measures to take these challenges into account. In case you’d like more information, please feel free to reach out to us.