In 2024, there is a feeling that AI is here to stay. Every software provider is trying, sometimes more and sometimes less sensibly, to incorporate AI into their own products. And initial results and studies show that the potential is enormous. But to get the most out of it, the right decisions need to be made and the use case, technology and people need to harmonise with each other.
One of the greatest potentials is the combination of grown, internal company data silos with generative AI in order to make the expertise that actually exists accessible to employees.
However, decision-makers are faced with the challenge of making the right decisions. Many companies are currently in a gold-rush mood and want to capitalise on the AI hype. For purchasing companies, it is a matter of making sensible long-term decisions in order to maintain an overview in the AI jungle.
This blog article serves as a guide for business leaders and IT decision-makers to develop a sensible basis for the introduction and use of AI in the company.
With insights into the technology, fields of application and a look at security aspects and people, this blog article provides a good guide for making a sustainable decision for your own company.
What is artificial intelligence?
Artificial intelligence (AI) is a generic term for all methods in which computers emulate human intelligence and cognitive abilities. AI is therefore not a specific technology, but a comprehensive category. You can think of it in a similar way to the term “communication”. Communication is the ability to exchange information, but does not yet say how this is done.
Everyone is currently talking about generative AI, but there are various types of AI.
One type of AI, for example, is machine learning. Whereas there used to be concrete, rule-based decision-making logic that could be used to solve predefined problems (e.g. pocket calculators), the processes are now much more complex. The challenge with rule-based software, however, is that tasks can only be completed if the logic has previously been written in programme code. Machine learning can be used to overcome this challenge.
Machine learning (ML) is a branch of artificial intelligence that uses algorithms and statistical models to enable computer systems to perform tasks without being explicitly programmed. The system uses information from past data as a basis for decision-making, which is taught to the AI in a so-called training process. The AI is then able to compare current information with the data, trends and patterns and make decisions based on this information.
Deep learning is a sub-category of machine learning.So-called neural networks – consisting of different “layers” – can recognise and learn even more complex patterns in large data sets. The “deep” in deep learning refers to the number of layers in the neural networks.
These systems are now so good that they can not only make decisions or individual predictions, but can also generate, process, shorten etc. entire texts. Such processing systems are then called large language models (LLMs).
Instead of actually generating texts, they calculate the next word based on a probability. This is how an AI-generated text is created.
And now the generation of such content is not just limited to text, but also extends to images, videos and audio.These techniques are then summarised as generative AI.
If you are wondering what an AI strategy for companies should take into account and what best practices have emerged in other companies, you should definitely read this blog article.
How large language models work
Ultimately, large language models (LLMs) are based on statistical probabilities that result from a previous learning process. Just as you probably think of the word “blue” at the beginning of the sentence “The sky is…”, an LLM continuously “estimates” the next likely matching (partial) word based on the previous text. In practice, this estimation process is repeated for each subsequent text segment until usable results, usually complete sentences, are obtained.
Using various techniques, it is possible to give AI models the ability to imitate tonality, personality, etc. This ensures intuitive and trustworthy results. This ensures an intuitive and trustworthy user experience and conveys the feeling of communicating with a real person.
Ultimately, however, large language models are just a technology and need to be well integrated into everyday life in order to realise added value.
“A software solution like amberSearch makes the added value of a technology like generative AI realisable for companies”
Bastian Maiworm, Managing Director amberSearch
At the bottom of the value chain (excluding hardware providers) are the AI model providers.
At the top are the end users (individuals or companies) who want to utilise the technology. In order to utilise the technology, however, software providers are required that enable meaningful and, above all, secure integration into existing IT systems, for example. Some providers, such as ChatGPT or OpenAI, train their own models and offer them to end users via a user interface.
Disadvantages of large language models
Readers of this blog post have probably also heard that training large language models (LLMs) is costly and resource-intensive. The learning process takes several months and requires a considerable amount of energy as well as high-performance hardware. According to current estimates, the cost of training OpenAI’s ChatGPT 4.0, for example, was around 100 million US dollars.Due to these high costs and the effort involved, the models cannot be continuously updated, but are generally based on the information that was available at the time of training.
AI models therefore have a static store of knowledge and have no direct connection to the internet. In order to make this information available to an AI model, a software manufacturer is required to establish this connection. Another weakness of LLMs is the phenomenon of hallucination. Due to the large amounts of data, the AI model sometimes “confuses” information from the different layers. The solution to this problem is explained later in the blog article.
If you are more interested in the technical finesse of generative AI in combination with internal company data, you should definitely read this blog article.
How companies can find the right use case for AI
In order to operate ever more efficiently, companies are striving for a high level of automation to facilitate scaling and reduce costs. Especially in the automation of communication and knowledge management, LLMs offer great potential and many new possibilities.
The great advantage of AI lies in the flexibility of LLMs. After all, specific software is no longer necessarily required to solve problems; instead, significantly more generic solutions such as amberSearch can be used to achieve (partial) automation, knowledge transfer and more.
Generative AI can, for example, help with analysing your own email inbox or creating contracts and presentations. But AI models with access to internal expertise can also be an important source of advice and inspiration in design or sales.
According to a study by Harvard Business School, employees who used a Large Language Model (LLM) to support them in their tasks were able to complete their tasks 25% faster and with 40% higher quality. This remarkable increase in productivity illustrates the immense potential of such technologies.
Given the wide range of possibilities, it is often difficult to identify the right use cases with the greatest potential. It is therefore important to start by gaining a centralised overview of the various possible uses of LLMs in companies. There are initially two broad core areas in which LLMs can be used:
Working with text: | Working with knowledge: |
– Creating new texts – Improving texts linguistically and stylistically – Summarising and paraphrasing texts – Analysing and interpreting texts – Combining and integrating different texts – Translating and localising texts | – Search large amounts of data for relevant information – Extract and present key messages and KPIs – Systematically structure knowledge and sources – Recognise correlations and patterns in data – Get quick and precise answers to complex questions – Predict forecasts and trends based on historical data |
*To avoid false expectations: Our amberSearch solution is not specialised in each of these areas. However, we are happy to provide further information about our solution in dialogue with you.
Examples from practice:
At amberSearch, we have now completed dozens of projects in the field of (generative) AI. Some of the use cases that are most interesting for companies are the following:
- Customer service – automation of answers to frequently asked questions through chatbots – either on the website for end users or service technicians who are at the customer’s site.
- Human resources – automated initial screening of CVs and preparation of interviews with applicants.
- Knowledge management – automatic structuring of existing documents and information, even over many years and across languages.
- Employees – answering recurring questions and supporting onboarding.
- Marketing – generation of creative content such as advertising copy, social media posts or blog articles.
- Sales – creation of personalised sales emails and chat support for sales staff. Internal research for references and customer communication
- Research & Search – finding relevant information faster in large amounts of data.
- Finance – analysing financial reports and generating report summaries.
- Software development – reviewing, correcting or generating code.
- Training and development – developing interactive and personalised learning materials and courses.
- Translation services – fast and cost-effective translation of documents or communications for international business.
- Data analysis – explaining complex data sets and summarising analyses in plain language.
- Email management – automating the prioritisation and response to incoming emails.
- Customer feedback analysis – extracting and analysing sentiment and opinions from customer reviews.
- IT Management – Automating the creation and management of documentation for software and systems.
- Contract management – automatically extracting information from contracts to monitor performance.
- Content curation – support in the selection and compilation of content.
If you’re wondering where to start, read our blog article “Identifying and prioritising AI use cases”. We have processed further valuable insights there.
Integration with internal data
Anyone who has read this far will have recognised this: In order to use the technology sensibly, it requires good integration into existing IT systems, which have often grown and consist of various (sometimes self-developed) data silos. And of course, these are not all available exclusively as cloud services; a lot of the information is also stored on-premise.
This is because most use cases require information from internal company content. Of course, users of the software could copy texts between the core applications such as Outlook, a DMS or a project management tool into the chat system. However, integration into existing systems would be much more practical – both in the user interface and with the systems’ databases.
The majority of AI projects will fail if either the integration into existing data silos is not sufficient (poor quality of responses) or the integration into existing user interfaces is not right (too little utilisation by users)
Bastian Maiworm, Managing Director amberSearch
A study by McKinsey found that there is enormous potential for increasing productivity, particularly when working with internal systems: Knowledge workers spend 1.8 hours a day searching for internal information. We have conducted similar surveys among our SME customers – but across all departments with computer workstations.
The result was daily search times of around 30 minutes – a figure that has risen by 77% in recent years and will continue to rise with increasing digitalisation. With 400 employees, a business case of over one million euros saved with the right tool can quickly be calculated.
Integration: how to combine internal data with AI
Now that a certain foundation has been laid and an understanding of the use of AI in the company has been created, we will now look at various options for integrating an LLM with your own data. Even if this chapter does not explicitly address GDPR and data protection-compliant implementation, concrete implementation under these guidelines would be mandatory.
Basically, the challenge today is not so much the technical integration of these systems. More important is the human factor, which should not be forgotten.
Option 1: Provide existing LLMs as a chatbot
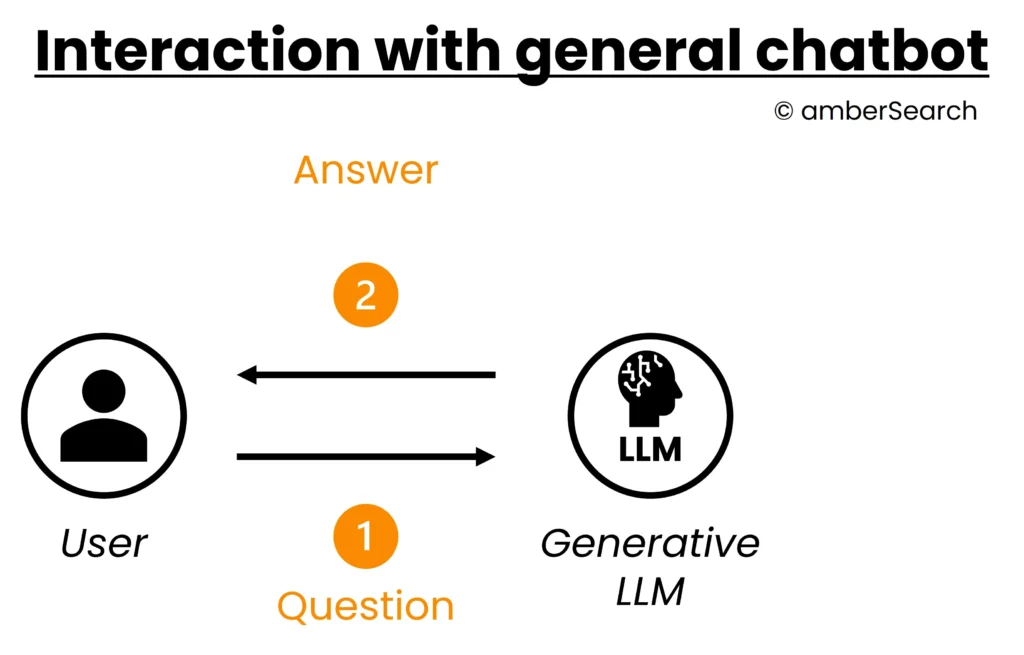
Visualisation of the interaction with a simple AI chat
The simplest integration into everyday working life is simply providing a chatbot as a user interface in your own systems – e.g. as a team app. If companies give their employees access to a chatbot, they have the option of copying information into it and having the chatbot carry out actions based on this. However, the LLM functions would then be a stand-alone solution and there would be no integration with the existing data silos. Even if this solution is very quick and easy to implement, it lacks all the monitoring and analysis options that are relevant for companies.
Option 2: AI functions integrated into existing systems
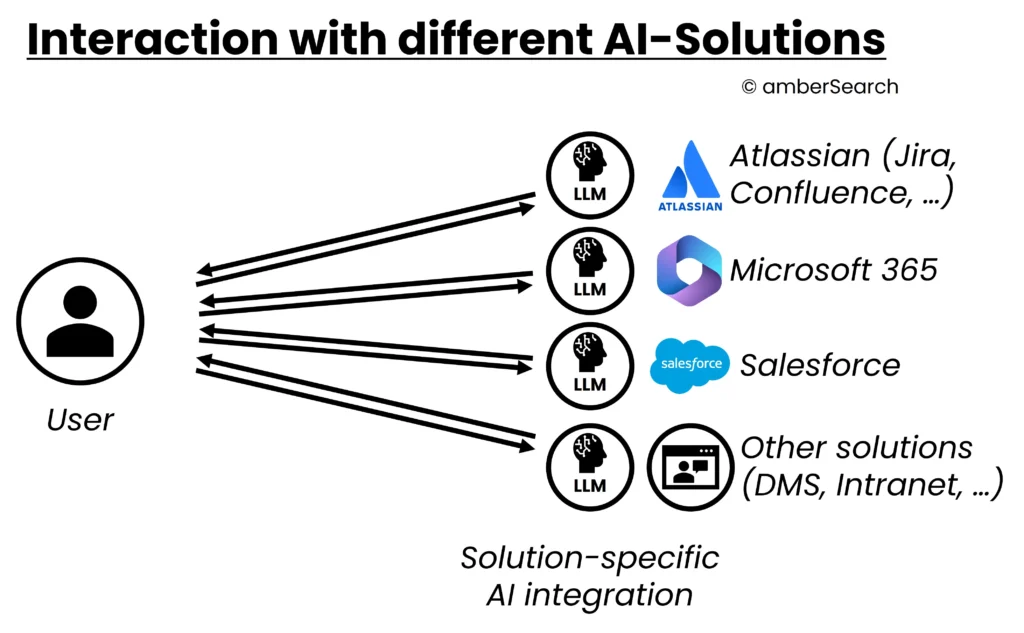
Illustration of an integration in which the native AI integration is used by each application
There is now hardly a software provider that does not develop its own AI. Businesses can use these deep integrations to chat with the system’s data. For example, a DMS provider could offer a “DMS-AI” with which you can then chat to DMS data. The advantage for users is clear: if the LLM is integrated directly into the respective business application, there is automatic data integration and there is no media discontinuity, as users do not have to switch between their usual app and a chatbot externally.
Even if this means deep integration into a system, the AI is still far from being integrated across all systems – although this is what most users expect. And sooner or later, procurement will also ask whether a separate AI system is necessary for each tool or whether all employees need access to it at high extra licence costs. This would lead to a further “fragmentation” of the IT landscape.
Option 3: Own LLMs or fine-tuning with own data
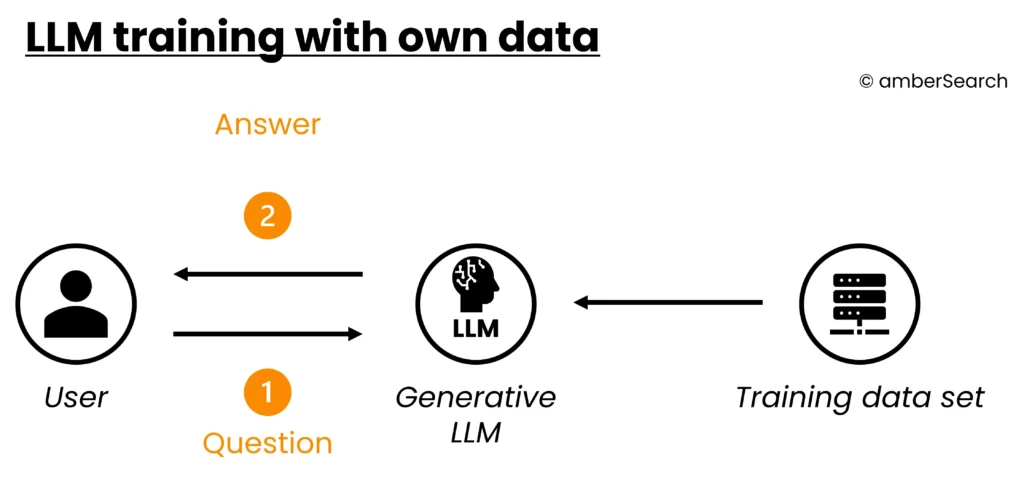
Illustration of the architecture of an LLM trained with own data
Instead of training their own AI model, companies can retrain or fine-tune an open source AI model. To do this, the company would have to provide a sufficient amount of its own data and use it to train the AI model. Even if this provides a high degree of independence and control, training and fine-tuning is still very expensive. There is also the challenge of hallucination and access rights. Furthermore, changing information in the company is not taken into account without re-training.
Option 4: AI assistants
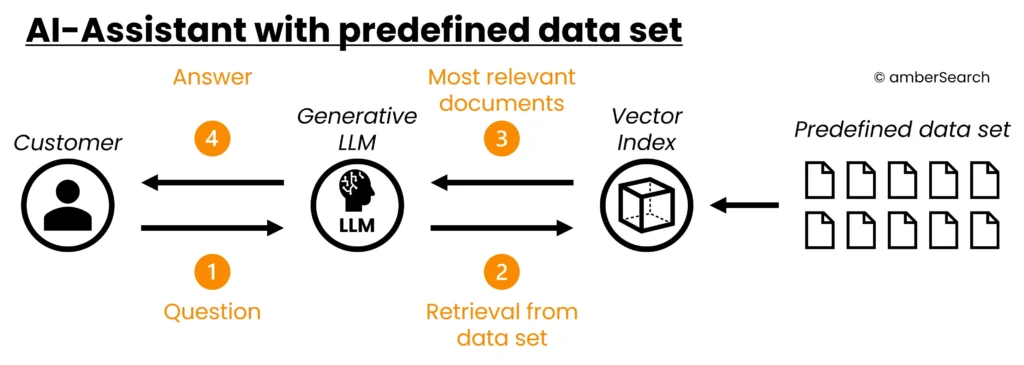
Illustration of the architecture of a Generative AI Search or an AI agent
A much better way of combining internal data with generative AI is to build an AI assistant. Once again, a data set must be defined as the basis for this. This could, for example, be an export of information on a specific topic. An index is then created from this data. If a user has a question, the AI assistant does not generate an answer based on the “learnt” information, but first searches the index for relevant information. These results are then used by the AI model to generate a suitable answer.
The AI model is basically told: “The user has asked question “X”. Results 1, 2 and 3 match the question well in terms of content. Use the content of these results to answer the user’s question.”. The advantage is that the index can be easily customised and thus changing data can be easily mapped. This approach also eliminates the problem of hallucination. The disadvantage is that there is still no deep integration with existing data and access rights are not taken into account. In addition, a separate AI assistant must be set up for each use case. This means that employees quickly lose the overview. The technology described here is called Retrieval Augmented Generation.
Option 5: AI agents or generative AI search
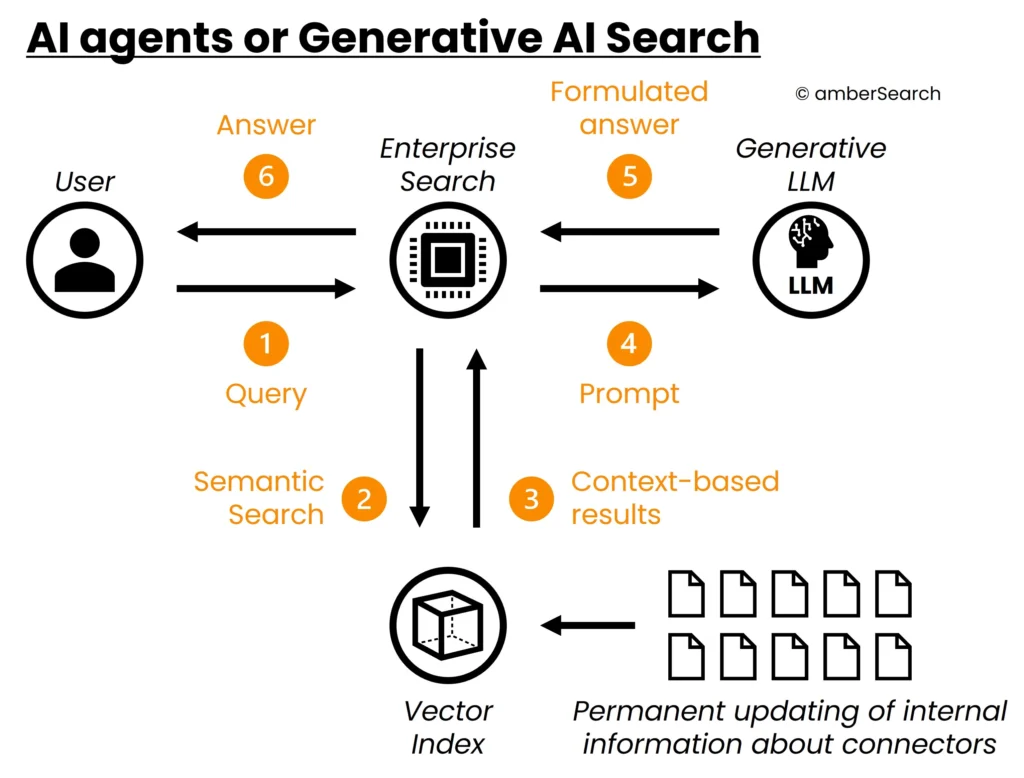
Illustration of the architecture of a Generative AI Search or an AI agent
The last, but also the most sustainable expansion stage for AI in the company is the introduction of AI agents or generative AI search. As this category is still relatively new, no clear definition has yet crystallised and many companies are trying to position themselves under this description, although they would not be according to our definition.
However, in order to achieve sustainable integration with internal systems, so-called connectors are required. These connectors can automatically create an index – taking access rights into account – and update it continuously. In this way, changing information can also be mapped by updating or deleting it in the index. However, because we are no longer talking about small, selected data records as with AI assistants, but rather the entire company knowledge is indexed, the index is not significantly larger.
This means that when processing a query, a powerful search must first be carried out using the index in order to utilise the most relevant results for the retrieval augmented generation system. An LLM-based enterprise search (company-internal search engine) is particularly suitable for this part, as it already has connectors to the various systems and specialises in searching large amounts of data. All data silos could be connected via the integration of such a software solution – taking access rights into account. With this technical approach, all internal data silos could be sustainably integrated into the IT infrastructure via an AI solution. The advantage is that a simple technical integration (less than 4 hours) of such a solution is possible via standardised connectors.
Security & data protection when using AI in companies
In this section, we would like to dedicate a separate section to the topic of security and data protection. For companies, the expertise that has been built up over the years is the most difficult to copy and is therefore particularly worth protecting. At the same time, the use of AI without a combination with internal data is of limited benefit.
Companies therefore rightly have reservations when it comes to using AI in combination with internal data in the company. In addition, customers are obliged to protect the privacy of their suppliers, customers and employees, which entails a further duty of care.
There is a persistent rumour circulating in German-speaking countries that all providers of large language models (LLMs) use user input data to further develop their models. This is not true. This practice only applies to the free and public versions of ChatGPT and similar models. When using business licences, the use of input data for training the models is excluded.
Interested companies should therefore ensure that they have the relevant documents, such as technical and organisational measures or server locations, explained to them and take a close look at the subcontractors in the order processing contract. To ensure sufficient security here, it is advisable to authorise or control AI tools centrally from IT and not allow employees to use their own tools. It is then no longer possible for companies to trace where their own data ends up.
Legally secure use of generative AI in the company
In order to be able to use AI solutions safely in the company, a few principles should be observed. This article explains what these are.
With amberSearch, we have built a solution that works completely independently of American providers and is fully GDPR-compliant for our customers, which range from SMEs to DAX-listed companies and from mechanical engineering to the critical infrastructure and pharmaceutical industries.
Introducing AI under the consideration of employees
In order to take a comprehensive look at the topic of AI, not only the technology, but also the employees should be brought on board. Many employees have not yet engaged with AI, do not understand the technology and believe, based on dangerous half-knowledge, that AI will replace us at some point. It is important to communicate proactively and create a safe framework in which employees can learn about AI and gain experience. If desired, we can provide support with our expertise.
In order to ensure that employees are taken on board, it is advisable to consider the steps recommended in this blog post.
Closing words
In this blog article, we have described many topics that touch on the introduction of generative AI with internal information. Companies are rightly asking themselves how they can realise the added value and where they should start. We hope that we have been able to provide a sufficiently in-depth insight into this topic with our expertise so that all points have been covered. Even if many companies start or have started with the integration of option 1, options 4 or 5 are much more sustainable in the long term. Most companies know that the development of a solution, especially as described under option 5, is not a case for in-house development due to the complexity of integration.
With amberSearch, we started developing our own retrieval models and connectors to the various data silos back in 2020. Today, this helps us to be the leading company in the segment of AI agents with internal company data. Customers from medium-sized companies, DAX-listed corporations and, for example, from the KRITIS or defence sector rely on our solution. We are happy to help with the introduction and implementation of an AI project.